Unlocking New Potential with RAG AI: From Data Retrieval to Intelligent Responses
Unlocking New Potential with RAG AI: From Data Retrieval to Intelligent Responses
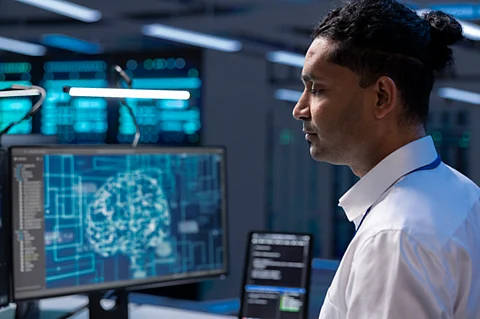
RAG (Retrieval-Augmented Generation) AI currently stands out for its ability to leverage external knowledge to produce smarter, contextually aware responses. By blending information retrieval with advanced generation techniques, RAG AI redefines the potential of conversational and problem-solving systems, providing accurate, data-backed answers in ways that traditional AI models can’t match.
Here, we’ll explore RAG AI meaning and how it is revolutionizing information processing, making it an indispensable tool across industries.
The Foundation of RAG AI: Retrieval Meets Generation
Retrieval-Augmented Generation (RAG) AI combines two essential components—data retrieval and text generation. Unlike traditional generative AI, which relies solely on a fixed set of trained data, RAG AI can dynamically pull in fresh information from a range of sources, like databases, APIs, or knowledge graphs, enhancing the model’s relevance and accuracy. This fusion allows it to answer highly specific questions based on real-time or continually updated data, making it a more flexible and powerful solution.
Real-Time Data Retrieval: A Key Differentiator
One of RAG AI’s key strengths lies in its ability to retrieve real-time information. When a user poses a question, RAG AI scans relevant data sources, gathers up-to-date information, and seamlessly integrates it into its response. This capability means that industries where information changes rapidly—such as finance, healthcare, or customer service—can rely on RAG AI to provide accurate insights and recommendations without the need for constant retraining.
Personalization and Context-Awareness
Another standout feature of RAG AI is its context awareness, which allows for hyper-personalized responses. By accessing data about individual users or specific environments, RAG AI tailors each response to the unique needs of the moment. For example, in e-commerce, RAG AI can recommend products based on recent trends, user preferences, or even regional availability, offering a level of personalization that enhances user satisfaction and engagement.
Enhancing Knowledge Management and Discovery
In knowledge-intensive sectors like law, research, and medicine, accessing the right data quickly can be critical. RAG AI supports these fields by improving knowledge management, allowing users to discover, retrieve, and apply information swiftly. With its ability to understand natural language and refine responses based on external databases or knowledge repositories, RAG AI minimizes the time spent on searching and synthesizing information, creating a more efficient workflow.
Scalability Across Various Industries and Use Cases
RAG AI’s flexibility has made it applicable across numerous industries. Here are a few examples of its impact:
Healthcare: RAG AI assists doctors by retrieving current medical research and patient records to deliver evidence-based treatment suggestions.
Financial Services: It enables analysts to make informed investment recommendations using real-time data on stock prices, market trends, and economic reports.
Customer Service: RAG AI enhances customer support interactions by pulling relevant information on product details, troubleshooting steps, or order status, streamlining the resolution process.
Reducing Data Silos and Boosting Interoperability
Organizations often struggle with data trapped in silos across departments. RAG AI can bridge these silos by pulling relevant information from multiple data sources, integrating it into a cohesive output. This interoperability strengthens decision-making by providing a holistic view of data that was previously dispersed, making it easier for teams to collaborate and act on unified insights.
Future Prospects: RAG AI as a Foundational Technology
The future of RAG AI is promising, as it evolves to support more complex and specialized queries, interact with various forms of media, and assist in predictive analytics. Future iterations may see it integrated with AI-driven analytics platforms, allowing it to not only retrieve and generate answers but also offer strategic insights based on predictive modeling. For companies aiming to stay competitive in the AI landscape, investing in RAG technology could yield a significant edge.
Discover What’s Next With RAG AI
Retrieval-Augmented Generation AI offers a glimpse into the future of intelligent information processing. With its unique ability to retrieve, synthesize, and generate contextually aware responses, RAG AI is reshaping how businesses and individuals interact with technology.
As it continues to advance, RAG AI is set to unlock even more possibilities—ushering in an era where intelligent responses are only the beginning.
Disclaimer: Analytics Insight does not provide financial advice or guidance. Also note that the cryptocurrencies mentioned/listed on the website could potentially be scams, i.e. designed to induce you to invest financial resources that may be lost forever and not be recoverable once investments are made. You are responsible for conducting your own research (DYOR) before making any investments. Read more here.
Artificial Intelligence
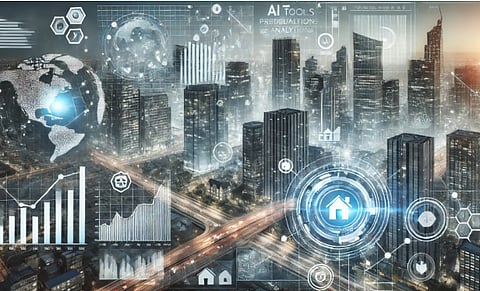
Harnessing the Power of Analytics and AI Tools For Real Estate Investments
IndustryTrends
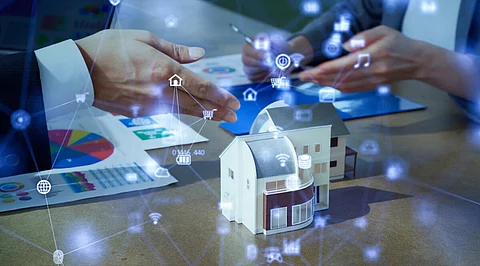
Maximizing Efficiency and Profits with the Best Address Verification Tools for the Real Estate Industry
IndustryTrends
How Edge Computing Is Enhancing AI Solutions
How to Drive More Revenue with Google Ads AI?
Artificial Intelligence
How Does Anthropic Computer Use Work?
The rapid evolution of AI has transformed the fabric of not only industries but also our societies. As AI becomes increasingly available, its impact on human values and sense of security is now a pressing concern. Amidst this, anthropic computing has now been seen as a promising approach to address the complex questions surrounding AI's influence on human values and well-being. By exploring this paradigm, we can better understand the intricate relationships between humans, technology, and society.
The design and development of the AI will be based on building systems that will support human values, safety, and well-being.
At the heart of anthropic computing is the idea of designing AI that aligns with human intentions and avoids causing unintended harm. It uses ethics, psychology, and cognitive science to create smart systems that tend toward human goals such as equity, transparency, and accountability. What it would mean is far more responsible and trusted AI, competent to make complex decisions by carefully considering its social and moral impact.
Human-centered design plays an important role in developing AI.
Anthropic computing, which correlates AI to humans' needs and values, provides the base of human-centric design. This method differs from traditional methods of artificial intelligence development, which often include giving a machine certain tasks to accomplish without regard to the effect the machine may create in human society through its decisions.
In anthropic computing, artificial intelligence makes decisions based on good human ethics and morals. Thus, AI systems have to solve problems, but they are also trained to do so in a manner that is morally right or socially responsible. For example, an AI designed to aid in medical diagnosis would be trained to prioritize patient safety, privacy, and dignity rather than mere efficiency or accuracy.
Stress on Safety and Trust in AI Systems
One of the primary goals of anthropic computing is to ensure that AI systems are safe for use in the real world. Improved capabilities raise concerns about possible misuse or impact that may result in AI because of actions that drones or an AI-powered autonomous car might have when not appropriately designed.
Anthropic computing addresses these concerns by focusing on safety protocols in AI and the ethics of its development. The bottom line is the prevention of bad consequences and accountability of AI systems toward their activities. Through this approach, developers seek to construct AI systems that not only perform efficiently but are also safe, trustworthy, and predictable.
Another vital aspect of anthropic computing is trust. The more reliance on AI in financial, health, and even educational institutions, the more transparent and explainable these systems must be in their decision-making mechanisms. This would bring transparency and trust in users' use of AI so that they understand how this AI makes decisions and, therefore, why a particular outcome can be reached.
The Role of Ethics and Accountability
Anthropological issues are critical in anthropic computing because AI systems are being implemented in high-stakes domains where decisions can lead to significant consequences, such as affecting credit scores, determining insurance premiums, or hiring or promotion. Without a framework for accountability and fairness, the chance of AI systems merely repeating existing biases or generating new forms of inequality exists.
Human-centred computing can look at these issues and bring ethics into the making of artificial intelligence. For example, algorithms should be fair and not biased, and it should be clear how an AI system operates. Developers and organizations using an AI system are responsible for accountability.
For example, if an AI system makes a prejudiced decision, then detection and correction mechanisms should exist to correct the errors. This could take place by auditing the decision process or by allowing users to dispute or appeal the decision. With a focus on ethics and accountability, anthropic computing aspires to create AI that not only functions well but is also just and responsible.
The Future of Anthropic Computing
Anthropocentric computing is one avenue in the development of AI. It's built around human values, with safety and ethics placed right up front in developing the systems themselves to best serve humanity's purposes. It has great promise at solving some of the immediate and fundamental questions facing the AI system—specifically, issues with respectability, fairness, privacy, or security. Demand for anthropic computing will increase with time as AI gets increasingly integrated into daily life.
All things considered, anthropic computing means the shift in the direction of more responsible and human-oriented AI. Ethics, safety, trust, and accountability would likely lead to a new form of future AI, ensuring that technology and humanity work in concert or according to a harmonious path by human values and purposes. As society will increasingly be more integrative with AI, then, by all means, the scope of anthropic computing becomes that much more pivotal.
Join our WhatsApp Channel to get the latest news, exclusives and videos on WhatsApp
Artificial Intelligence
Caught in a Data Trap? What to Do if Your Information is Stolen
How to Register and Login to HDFC Net Banking?
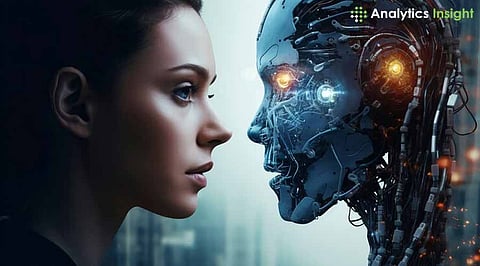
Inside the World of Self-Evolving AI Systems
Artificial Intelligence
Decentralized Data Collection: What Does It Even Mean?
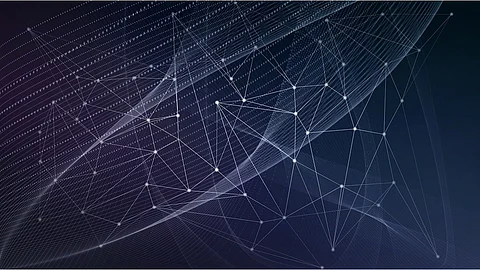
Applications utilizing Artificial Intelligence (AI) require large data sets for training to ensure they're accurate and can handle diverse scenarios. Unfortunately, traditional data collection processes have many hurdles to overcome, which can reduce efficiency, increase costs, and lower data quality.
However, decentralized data collection presents a solution. It is set to revolutionize the industry by merging data collection with an immutable distributed ledger known as the blockchain. This merging of technologies will enable participants to work together without a central authority, providing manipulation resistance and enhanced privacy.
Now that we have a rough idea of the limitations present in the data collection space and how a decentralized approach could help overcome them, let’s dive deeper.
What’s Slowing Down Data Collection and Labelling Nowadays?
There are several areas where traditional data collection methods lack effectiveness and could cause issues when used for models with real-world applications:
Data Manipulation
The manipulation of data is a substantial issue in traditional systems. Whether it’s bad actors intentionally providing false data, AI-generated content affecting data, or malicious attacks designed to impact model performance, manipulation in current data collection processes can lead to significant losses and time-consuming problems.
Diversity and Volume of Data
Data collection in the current sense often experiences bias as systems can underrepresent specific demographics, which can lead to lackluster performance in real-world settings. Additionally, large data sets usually come with significant costs that can price out small start-up projects, making the need for a more cost-effective solution apparent.
Privacy and Ethical Concerns
Current data collection systems struggle to comply with privacy regulations like the General Data Protection Regulation (GDPR), which can lead to legal concerns. According to a report from Pew Research, up to 73% of Americans feel they have no control over how their data is used, which creates a division between consumers and companies.
Quality of Data
Maintaining data quality is critical to train AI models effectively. However, factors like bias, issues with labeling, and redundant information in data sets reduce quality. As a result, they require additional processing power to validate or clean, which leads to a notable increase in costs.
Here’s How The Decentralized Approach Comes Into Play
Decentralized data collection tools integrate the blockchain, a technology common in cryptocurrency projects, to act as a secure record of transactions and information. It is immutable and cannot be manipulated, making it far easier to identify fabricated data and helping to improve overall data quality and model performance.
The integration of the blockchain results in a distributed network of devices referred to as nodes, which are responsible for storing and managing data. Each node can make decisions regarding the authenticity and availability of data independently without relying on a central authority, which promotes greater efficiency.
On top of improving efficiency, integrating the blockchain has additional benefits. Instead of gathering data from a single provider, decentralized systems democratize data sourcing, enabling businesses to collect information directly from participants. This approach has key advantages, such as lowered costs, improved privacy, and increased quality.
OORT, for example, a cloud for decentralized AI, offers a community-powered data collection service dubbed “DataHub”. By utilizing a decentralized network, OORT’s DataHub can eliminate intermediaries, which enhances security, transparency, and participant control in large-scale data collection and labeling.
Image source: OORT DataHub
The service incentivizes people from around the world to contribute to data collection. This results in a broader pool of sources, which helps OORT DataHub create diverse and representative data sets geared to real-world use cases. By catering to worldwide users, this approach facilitates global participation at lower prices and will help advance AI and machine learning model training in a cost-effective way.
Through its use of blockchain technology, OORT DataHub can reduce the risks of manipulation, helping it foster a transparent and fair foundation for AI development. Decentralized systems like this are paving the way for ethical data collection in artificial intelligence and help to keep the industry socially responsible and aligned with users' best interests.
Web Site : sensors.sciencefather.com
Visit Web Site : sciencefather.com
Nomination Link : https://sensors-conferences.sciencefather.com/award-nomination/?ecategory=Awards&rcategory=Awardee
Contact as : sensor@sciencefather.com
Social Media
Twitter :https://x.com/sciencefather2
Pinterest : https://in.pinterest.com/business/hub/
Linkedin : https://www.linkedin.com/feed/
#ScienceFather, #Researchaward,#ArtificialIntelligence, #AI, #MachineLearning, #DataScience, #BigData, #DeepLearning, #NLP, Natural Language Processing #ClimateResilience, #GreenAgriculture, #FoodSecurity, #SustainableDevelopment, #AgriTech, #RegenerativeFarming, #CarbonSequestration, #ClimateAction, #ResilientFarming, #PrecisionAgriculture, #SmartFarming, #Agroecology, #SoilHealth, #WaterManagement, #AdaptationAndMitigation, #EcoFriendlyFarming, #AgriculturalSustainability #EnvironmentalSafety, #MiningHealth, #ConfinedSpaceSafety, #SustainableVentilation #DigitalHealth #PrincipalInvestigator, #ClinicalResearchCoordinator, #GrantWriter, #R&DManager, #PolicyAnalyst, #TechnicalWriter, #MarketResearchAnalyst, #EnvironmentalScientist, #SocialScientist, #EconomicResearcher, #PublicHealthResearcher, #Anthropologist, #Ecologist,
Comments
Post a Comment