The Power of Computer Vision in AI: Unlocking the Future!
The Power of Computer Vision in AI: Unlocking the Future!
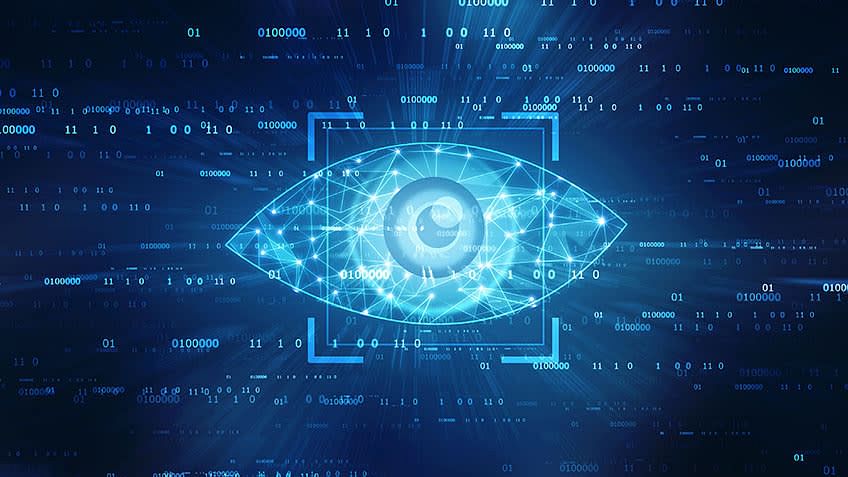
Human vision extends beyond the mere function of our eyes; it encompasses our abstract understanding of concepts and personal experiences gained through countless interactions with the world. Historically, computers could not think independently. However, recent advancements have given rise to computer vision, a technology that mimics human vision to enable computers to perceive and process information similarly to humans.
Computer vision has witnessed remarkable advancements fueled by artificial intelligence and computing capabilities breakthroughs. Its integration into everyday life is steadily increasing, with projections indicating a market size nearing $41.11 billion by 2030 and a compound annual growth rate (CAGR) of 16.0% from 2020 to 2030.
What Is Computer Vision?
Computer vision is an artificial intelligence domain instructing computers to comprehend and interpret visual data. Leveraging digital images sourced from cameras and videos, coupled with advanced deep learning algorithms, computers adeptly discern and categorize objects, subsequently responding to their visual environment with precision.
Key Aspects of Computer VisionImage Recognition: It is the most common application, in which the system identifies a specific object, person, or action in an image.
Object Detection: This involves recognizing multiple objects within an image and identifying their location with a bounding box. This is widely used in AI applications such as self-driving cars, where it’s necessary to recognize all relevant objects around the vehicle.
Image Segmentation: This process partitions an image into multiple segments to simplify or change the representation of an image into something more meaningful and easier to analyze. It is commonly used in medical imaging.
Facial Recognition: This is a specialized application of image processing where the system identifies or verifies a person from a digital image or video frame.
Motion Analysis: This involves understanding the trajectory of moving objects in a video, commonly used in security, surveillance, and sports analytics.
Machine Vision: This combines computer vision with robotics to process visual data and control hardware movements in applications such as automated factory assembly lines.
How Does Computer Vision Work?
Computer vision enables computers to interpret and understand digital images and videos to make decisions or perform specific tasks. The process typically starts with image acquisition, capturing visual data through cameras and videos. This data then undergoes preprocessing, including normalization, noise reduction, and conversion to grayscale to enhance image quality. Feature extraction follows, isolating essential characteristics such as edges, textures, or specific shapes from the images. Using these features, the system performs tasks like object detection (identifying and locating objects within the image) or image segmentation (dividing the image into meaningful parts).
Advanced algorithms, particularly Convolutional Neural Networks (CNNs), are often employed to classify and recognize objects accurately. Finally, the analyzed data can be used to make decisions or carry out actions, completing the computer vision process. This enables applications across various fields, from autonomous driving and security surveillance to industrial automation and medical imaging.
Image Analysis Using Computer Vision
Image analysis using computer vision involves extracting meaningful information from images through various computational techniques. This process is fundamental in numerous applications across multiple industries, including healthcare, automotive, security, and entertainment. Here’s a breakdown of how image analysis is typically conducted using computer vision technologies:
1. Image Preprocessing
Before analysis, images often undergo preprocessing to improve their quality and enhance important features for further image processing. Common preprocessing steps include:Grayscale Conversion: Reducing the image to grayscale to simplify the analysis by eliminating the need to process color.
Noise Reduction: Applying filters to smooth out the image and reduce noise that could interfere with analysis.
Normalization: Adjusting the pixel intensity for uniformity.
Edge Detection: Highlighting the edges in the image to better define boundaries and shapes.
2. Feature Extraction
Feature extraction involves identifying and isolating various characteristics or attributes of an image. Features might include shapes, textures, colors, or specific patterns. Effective feature extraction is crucial as it directly influences the accuracy and efficiency of the subsequent analysis phases.
3. Segmentation
Segmentation divides an image into multiple segments (sets of pixels, also known as superpixels) to simplify and change the representation of the image into something more meaningful. There are different methods of segmentation:Thresholding: Separating pixels based on a predefined criterion.
Region-based Segmentation: Dividing an image into regions according to predefined criteria.
Edge-based Segmentation: Detecting edges to find boundaries.
Clustering: Grouping pixels into clusters based on similarity.
4. Object Detection and Recognition
This step involves identifying objects within an image and classifying them into known categories. This can be achieved through various methods:Template Matching: Comparing different parts of an image to a template to detect the presence of specific objects.
Machine Learning: Using trained algorithms to recognize objects. This typically involves training a model on a large dataset with labeled images.
Deep Learning: Applying Convolutional Neural Networks (CNNs) that can automatically detect and classify various objects in an image with high accuracy.
5. Analysis and Interpretation
After detecting and classifying objects, the system analyzes the context or changes over time (in the case of video) to derive insights. This step might involve:Pattern Recognition: Identifying patterns or anomalies within an image.
Statistical Analysis: Calculating various statistics, like object counts or size distributions.
Machine Vision: Interpreting images to guide action (e.g., in robotic process automation).
6. Decision Making
The final step involves making decisions based on the analyzed data. This can range from triggering an alert when a certain object is detected to providing diagnostic insights in medical imaging.
WEBSITE : sensors.sciencefather.com
Nomination Link : https://sensors-conferences.sciencefather.com/award-nomination/?ecategory=Awards&rcategory=Awardee
Registration Link :https://sensors-conferences.sciencefather.com/award-registration/
contact as : sensor@sciencefather.com
SOCIAL MEDIA
Twitter :https://x.com/sciencefather2
Blogger : https://x-i.me/b10s
Pinterest : https://in.pinterest.com/business/hub/
Linkedin : https://www.linkedin.com/feed/
#sciencefather #researchaward #EdgeComputing, #IIoT, #IndustrialIoT, #SmartManufacturing, #DigitalTransformation, #IoT, #ManufacturingTech, #Industry40, #DataProcessing, #Automation, #TechTrends, #IndustrialAutomation #Lecturer, #Scientist, #Scholar, #Researcher, #Analyst, #Engineer, #Technician, #Coordinator, #Specialist, #Writer, #Assistant, #Associate, #Biologist, #Chemist, #Physicist, #Statistician, #DataScientist, #Consultant, #Coordinator, #ResearchScientist, #SeniorScientist, #JuniorScientist, #PostdoctoralResearcher, #LabTechnician, #ResearchCoordinator, #PrincipalInvestigator, #ClinicalResearchCoordinator, #GrantWriter, #R&DManager, #PolicyAnalyst, #TechnicalWriter, #MarketResearchAnalyst, #EnvironmentalScientist, #SocialScientist, #EconomicResearcher, #PublicHealthResearcher, #Anthropologist, #Ecologist,
Comments
Post a Comment