Sensors take center stage for moving Gen AI to cars, robots
Sensors take center stage for moving Gen AI to cars, robots
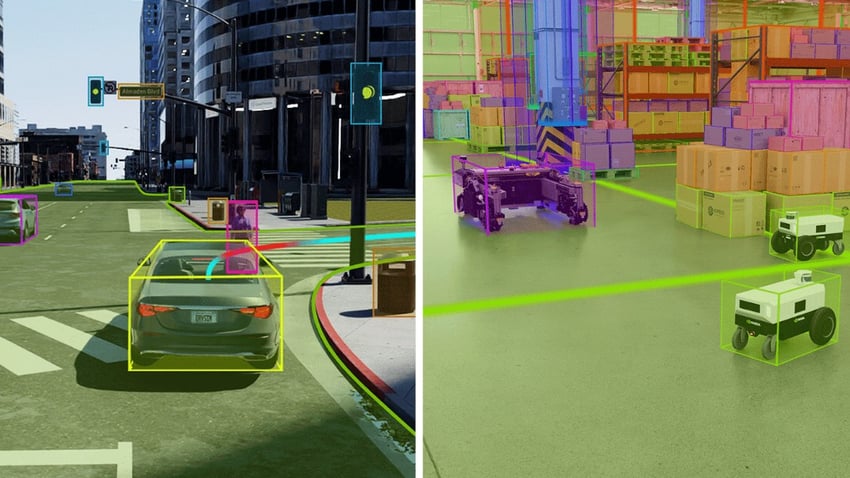
This story has been updated from an original version that ran June 17.
Generative AI has energized industries of all types in many ways: for building better customer service (better chatbots, for example) and organizing data across industries like finance and marketing. It has even given coders shortcuts for building better programs, much less helping administrators in writing reports with predictive capabilities.
One area that has received less attention, however, is using GenAI to to build applications for use cases in the generative physical AI realm (yes, some analysts are using that term). These include robotics, especially automobiles viewed as a type of robot, and for industrial machinery.
It turns out that sensors, a multibillion-dollar industry, are at the center of this movement (obviously, but maybe not so obviously), where autonomous vehicles, humanoid and mobile robots and industrial machines will use data from sensors to make sense of the physical world. Nvidia CEO Jensen Huang talked about Gen AI for machines of all types at GTC earlier this year, but now the company is being more specific and concrete about how it intends to move forward.
RELATED: Huang posits Nvidia has moved well beyond GPUs, but isn’t a CSP
On Monday, Nvidia introduced a set of microservices available later this year called Nvidia Omniverse Cloud Sensor RTX, where developers can test sensor perception alongside its associated AI software and at scale in physically accurate, realistic virtual environments prior to real-world deployment. Nvidia named Foretellix and MathWorks as among the first to receive the software. The microservices are cloud-native and can be self-deployed on a private cloud or a public cloud, Nvidia told Fierce. (HPE also separately announced a private AI cloud powered by Nvidia.)
The rollout seems like an obvious direction, but one that Nvidia is emphasizing in its increasingly expanding product set that is best known for its mega accelerating GPUs like Hopper and the upcoming Blackwell platform. Omniverse has already been around for years, along with Nvidia’s work in promoting the use of digital twins, but the sensor part is what’s somewhat new in its formal role in Sensor RTX, although advanced development teams in various industries are already doing such work on their own.
“Developing safe and reliable autonomous machines powered by generative physical AI requires training and testing in physically-based virtual worlds,” said Rev Lebaredian, vice president of Omniverse and sim technology at Nvidia, in a prepared statement. “Nvidia Omniverse Cloud Sensor RTC microservices will enable developers to easily build large-scale digital twins of factories, cities and even Earth—helping accelerate the next wave of AI.”
The main thing Omniverse Cloud Sensor RTX offers developers is acceleration in their work creating simulated environments. That means combining real-world data from videos, cameras, radar and lidar with synthetic data. Conceivably this also means, at some point, all the sensors used in flexible fabrics for medical applications, or room sensors used to evaluate crowd movements and temperature, pressure and gasses. There are many possibilities.
Nvidia said its microservers can be used to simulate various activities such as whether a robotic arm is operating properly, an airport luggage carousel if functional, a tree branch is blocking a roadway, a conveyor belt is in motion or a robot or person is nearby. Amazon has already done similar work using its own design processes with mobile robots in sorting warehouses, while John Deere has been slowly rolling out autonomous tractors that sense a fallen tree or another object then bring the tractor to a stop or find a new direction.
For years, John Deere developed the code to help train its tractors to “recognize” a person or animal running across the field to then take action on the tractor’s drive train. At one point, the company even hired actors to cross a field, so the system could be trained to recognize what a person crossing the field looks like to a machine. All the major car companies have spent years developing different methods of converting sensing in modern vehicles (sometimes with dozens of sensors per vehicle) into intelligible data with commands for autonomous vehicle movements. Compared to the years of development work by such companies, the Nvidia approach could reduce the time needed for physical prototyping, although it is still early in the rollout process to judge.
The new software is a “scaled-down Omniverse for IoT based on an RTX engine and available microservices, which makes it more available for smaller scale environments,” said Jack Gold, founding analyst at J. Gold Associates. He said the usefulness of the software will depend on how specific the microservices are and many IoT development projects are “pretty unique.” However, he said many visual tasks are similar to one another, which means Omniverse Cloud Sensor RTX could be a “good starter platform.”
Patrick Moorhead, chief analyst at Moor Insights & Strategy, said the Omniverse Cloud Sensor RTX basically takes what Nvidia has been doing in the automotive space and extends it to different areas. "The concept is similar across all use cases which is to speed up training to have more accurate results," he said. More importantly, Nvidia is relying on the OpenUSD standard, which is designed to ultimately speed up training and accuracy.
Nvidia's Cloud Sensor RTX announcement came just three days after Nvidia released an open synthetic data generation pipeline to train LLMs called Nemotron-4 340B, which work with Nvidia NeM and Nvidia Tensor RT-LLM.
Cloud Sensor RTX was announced at the Computer Vision and Pattern Recognition conference in Seattle where Nvidia also submitted research, including two papers in training dynamics of diffusion models and high def maps for AVs that were named as finalists in CVPR’s Best Paper Awards.
Editor’s Note: The convergence of sensors and AI has been happening across industries and will be a feature of Sensors Converge 2024, which runs June 24-26 in Santa Clara. Registration is online. Use the code HAMBLEN at registration to get a free Expo Hall pass or save up to $200 on a VIP or Conference Pass.
WEBSITE : sensors.sciencefather.com
Nomination Link : https://sensors-conferences.sciencefather.com/award-nomination/?ecategory=Awards&rcategory=Awardee
Registration Link :https://sensors-conferences.sciencefather.com/award-registration/
contact as : sensor@sciencefather.com
SOCIAL MEDIA
Twitter :https://x.com/sciencefather2
Blogger : https://x-i.me/b10s
Pinterest : https://in.pinterest.com/business/hub/
Linkedin : https://www.linkedin.com/feed/
#sciencefather #researchaward#Sensors, #AI, #Robotics, #AutonomousVehicles, #GenAI, #MachineLearning, #SmartTechnology, #ArtificialIntelligence, #IoT, #Automation, #TechInnovation, #SelfDrivingCars, #FutureTech, #SmartRobots, #AIinAutomotive, #AIinRobotics, #SensorTechnology, #AIRevolution, #AutomotiveTech, #RobotSensors, #AdvancedSensors #ResearchCoordinator, #PrincipalInvestigator, #ClinicalResearchCoordinator, #GrantWriter, #R&DManager, #PolicyAnalyst, #TechnicalWriter, #MarketResearchAnalyst, #EnvironmentalScientist, #SocialScientist, #EconomicResearcher, #PublicHealthResearcher, #Anthropologist, #Ecologist,
Comments
Post a Comment